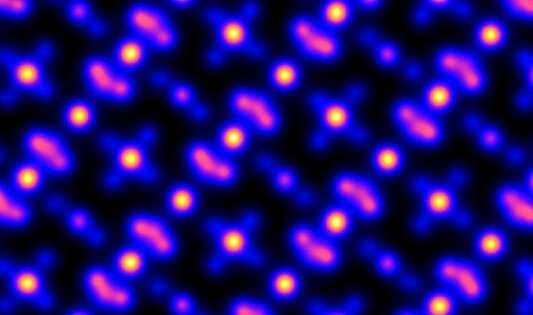
- 27-04-2022
- MACHINE LEARNING
A team of researchers from the USA developed and demonstrated two new techniques for better understanding the inverse problems.
Supercomputers help to study the causes and effects—usually in that order—of complex phenomena. However, scientists occasionally need to deduce the origins of scientific phenomena based on observable results. These so-called inverse problems are notoriously difficult to solve, especially when the amount of data that must be analyzed outgrows traditional machine-learning tools.
To reduce coordinator-worker communication, which often involves repeating the same requests multiple times, the team introduced a response cache that stores the metadata from each request. The first new strategy was this caching approach, which allows to immediately recognize and automatically calculate familiar requests.
The second new technique involves grouping the mathematical operations of multiple DNN models, which streamlines tasks and improves scaling efficiency—the total number of images processed per training step—by taking advantage of the similarities in each model's calculations. This process leads to significant improvements in power usage as well.
By strategically grouping these models, the team aims to eventually train a single model on multiple GPUs and achieve the same efficiency obtained when training one model per GPU.