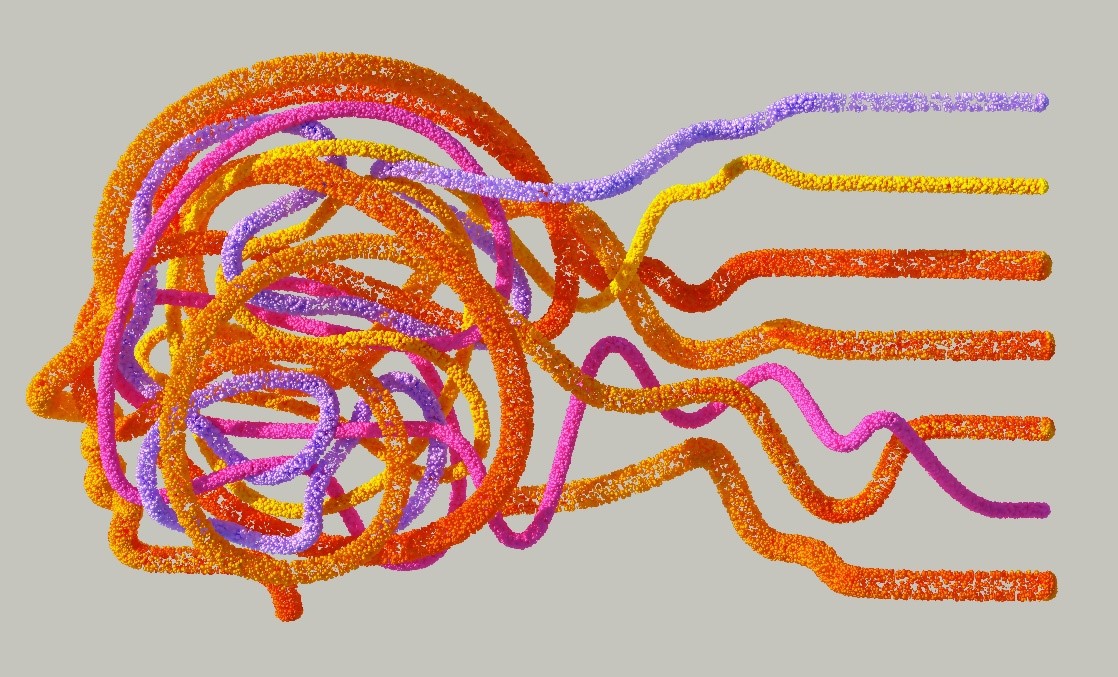
- 09-05-2025
- Artificial Intelligence
AI's rationality should be prioritized over intelligence, focusing on improving decision-making and aligning with human reasoning, to enhance AI's effectiveness in real-world applications.
A recent article explores the critical question of whether AI is more rational than humans, rather than simply smarter. The piece argues that while advanced AI systems like GPT-4 demonstrate impressive cognitive abilities, they often outperform human reasoning in certain contexts but fail to fully replicate the complexities of human decision-making.
AI’s potential lies in its ability to enhance rationality, not just intelligence. By understanding and incorporating human decision-making patterns, AI systems can become more effective, complementary tools for solving real-world problems.
The article underscores the importance of focusing on the rationality of AI, suggesting that this approach could lead to more intuitive and adaptive systems, ultimately improving their integration into everyday applications.