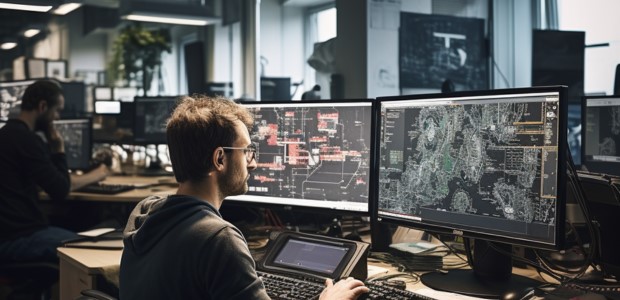
- 27-01-2024
- DATA SCIENCE
Researchers explore multi-attacks on image classification systems. They introduce a new optimization techniques, emphasizing the need for enhanced AI defense against adversarial threats.
Researchers at Stanislav Fort delve into the alarming realm of multi-attacks on image classification systems, uncovering vulnerabilities beyond current defense strategies. Their innovative methodology employs standard optimization techniques, rooted in a toy model theory, utilizing the Adam optimizer in machine learning. The approach not only executes successful multi-attacks but also delves into understanding the pixel space landscape for optimal manipulation. Results emphasize the complexity of class decision boundaries and raise concerns about AI training practices. The study underscores the growing importance of fortifying AI against adversarial threats as its integration across industries accelerates, urging advancements in image classification models' security.