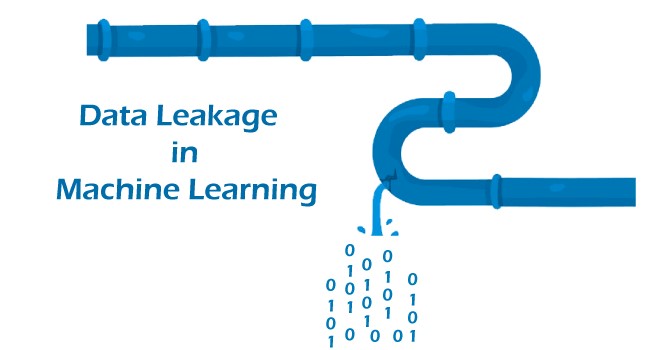
- 06-03-2024
- Machine Learning
A Yale study highlights data leakage's disruptive impact on machine learning models, distorting predictions. Vigilance in prevention is crucial for maintaining model reliability.
In a study published in Nature Communications, Yale University researchers investigated how data leakage affects machine learning models. Data leakage, where information from the testing dataset influences model training, can distort results. They found that leakage can inflate the model's prediction performance, particularly through "feature selection" and "repeated subject" leakage types. This inflation can mislead researchers into believing the model performs well when it struggles with truly unseen data. Furthermore, leakage effects are more pronounced in smaller sample sizes. To mitigate this, researchers advocate for transparency, sharing code, and maintaining a healthy skepticism about results. By avoiding data leakage, the reliability and reproducibility of machine learning models can be ensured.