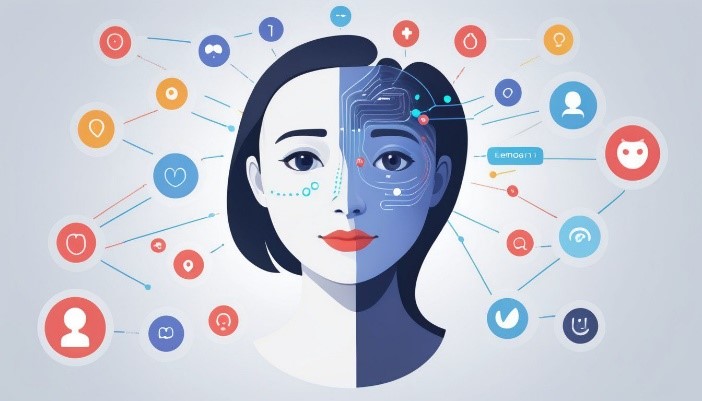
- 11-10-2024
- Artificial Intelligence
AI detects emotions in social media posts, influencing donation behavior and offering marketing insights. However, this raises privacy and ethical concerns.
Recent research published in the International Journal of Market Research reveals that AI can accurately detect specific human emotions in social media posts, particularly on X (formerly Twitter). By using a transformer transfer learning model, the study analyzed over 3.6 million sentences to identify emotions like joy, anger, sadness, and disgust. The findings show that emotions expressed in tweets significantly influence donation behavior toward non-profit organizations, with sadness driving donations to the Fred Hollows Foundation and anger increasing contributions to the University of Auckland. While this capability has implications for marketing and decision-making, it raises ethical concerns about privacy and responsible usage as AI continues to evolve in understanding human emotions.