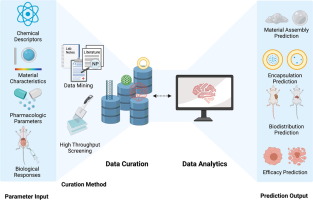
- 12-06-2024
- Artificial Intelligence
Researchers have developed an automated data curation technique that matches or surpasses manual curation for AI training, enhancing efficiency and accuracy.
A team of researchers from FAIR at Meta, INRIA, Université Paris Saclay, and Google have developed an automated technique for data curation that enhances self-supervised pre-training of AI datasets. This new method involves a three-step process: using a feature-extraction model to embed data points, applying successive k-means clustering to group similar data points, and employing multi-step hierarchical k-means clustering to ensure balanced data clusters. Testing with vision models showed that their technique performed as well as, or better than, manual curation, highlighting its potential for improving AI training efficiency and accuracy. Further testing is needed to evaluate its effectiveness on real-world data and various AI systems.