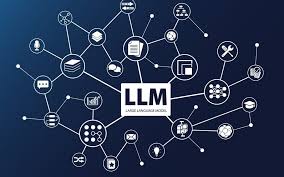
- 31-01-2025
- LLM
Transformer2 is a self-adaptive LLM that dynamically adjusts its weights for new tasks, improving flexibility eliminating the need for traditional fine-tuning.
Transformer2 is a self-adaptive LLM designed to dynamically adjust its weights when faced with new tasks, eliminating the need for traditional fine-tuning. Unlike conventional models, which require parameter adjustments followed by retraining, Transformer2 adapts on the fly as it encounters unfamiliar tasks.
The model works in two stages. First, it analyzes the incoming task to determine the necessary response. Then, it adjusts its internal weight system using Singular Value Decomposition (SVD) and reinforcement learning, helping it prioritize the most relevant information for the task at hand.
During inference, Transformer2 employs three main strategies: prompt-based, classifier-based, and a few-shot adaptation method. This flexibility allows the model to perform well on both familiar tasks and new, challenging queries, making it more versatile than traditional LLMs. The result is an AI that can adjust to different types of tasks dynamically, offering improved efficiency and flexibility.