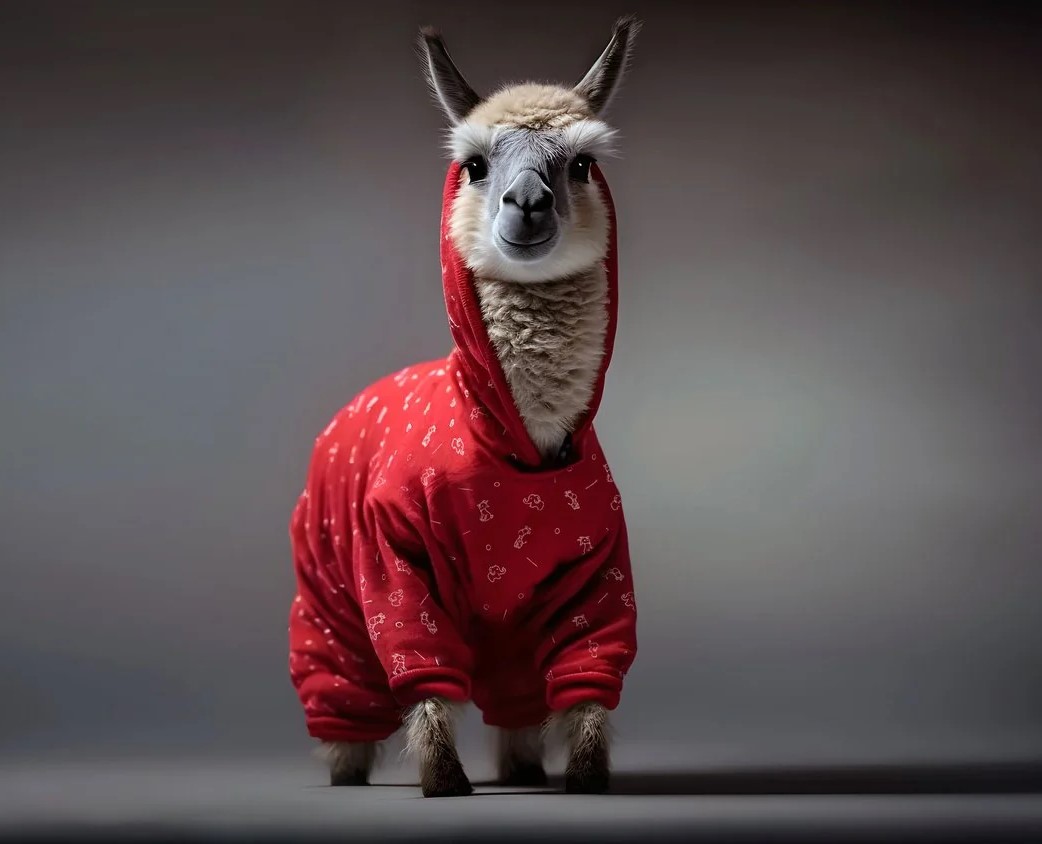
- 08-11-2023
- Data Analytics
Explore the intricacies of Density Kernel Depth (DKD), a groundbreaking method for outlier detection in functional data, providing clarity in complex datasets and addressing unique challenges.
The Density Kernel Depth (DKD) method emerges as a crucial tool for outlier detection in functional data, addressing the challenges posed by complex curves and surfaces. Unlike traditional approaches suited for scalar or multivariate data, functional data, represented by continuous curves, requires advanced techniques. DKD compares the density of each curve to the overall dataset density using non-parametric kernel methods. This yields a depth value for each curve, facilitating efficient outlier detection. DKD's flexibility, interpretability, and computational efficiency make it suitable for diverse functional data structures. In practical scenarios, such as analyzing patient heart rate curves, DKD offers a holistic perspective, detecting entire abnormal curves for more comprehensive insights. As data complexity grows, DKD exemplifies a promising tool for navigating intricate functional data landscapes, paving the way for sophisticated analysis techniques.