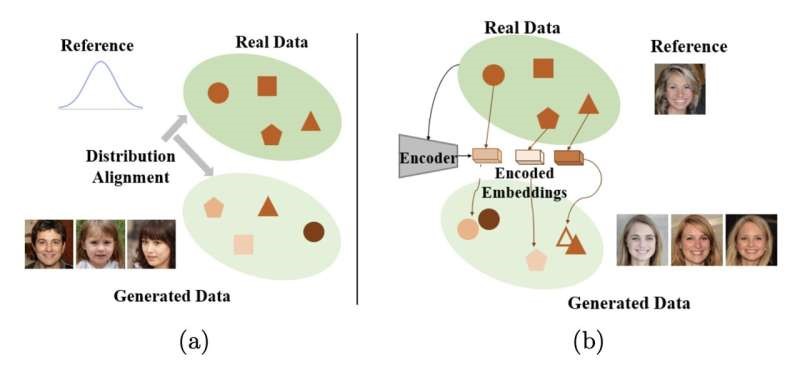
- 13-12-2024
- AI
A new image generation framework allows researchers to control content by using reference images, improving content alignment and generating images with 85% content accuracy..
The newly developed image generation framework by researchers from Seoul National University of Science and Technology addresses the challenge of controlling the content in generated images, a common limitation of traditional generative models like GANs. While GANs often produce random synthetic images, the new framework allows users to specify desired content by using a reference image. This content-preserving process is achieved through advanced encoding techniques, which involve two main components: the frequency encoding module and the content fusion module.
The frequency encoding module captures key features and structures from the reference image, focusing on specific frequency components, while the content fusion module creates a guiding vector that encapsulates the desired content features. These content-guiding vectors are then fused with projected noise vectors during the image generation process, ensuring that the output maintains the content of the reference image but introduces stylistic variations.
In tests, the framework outperformed conventional GAN models by generating images that better aligned with the reference image, retaining about 85% of its key attributes. This innovative framework shows promise not only for generating highly tailored datasets to train computer vision models but also for creative fields, such as design, where precise control over generated content is crucial. The ability to create images that meet specific expectations opens new possibilities for AI-assisted content creation and design, offering a significant improvement over current generative models.